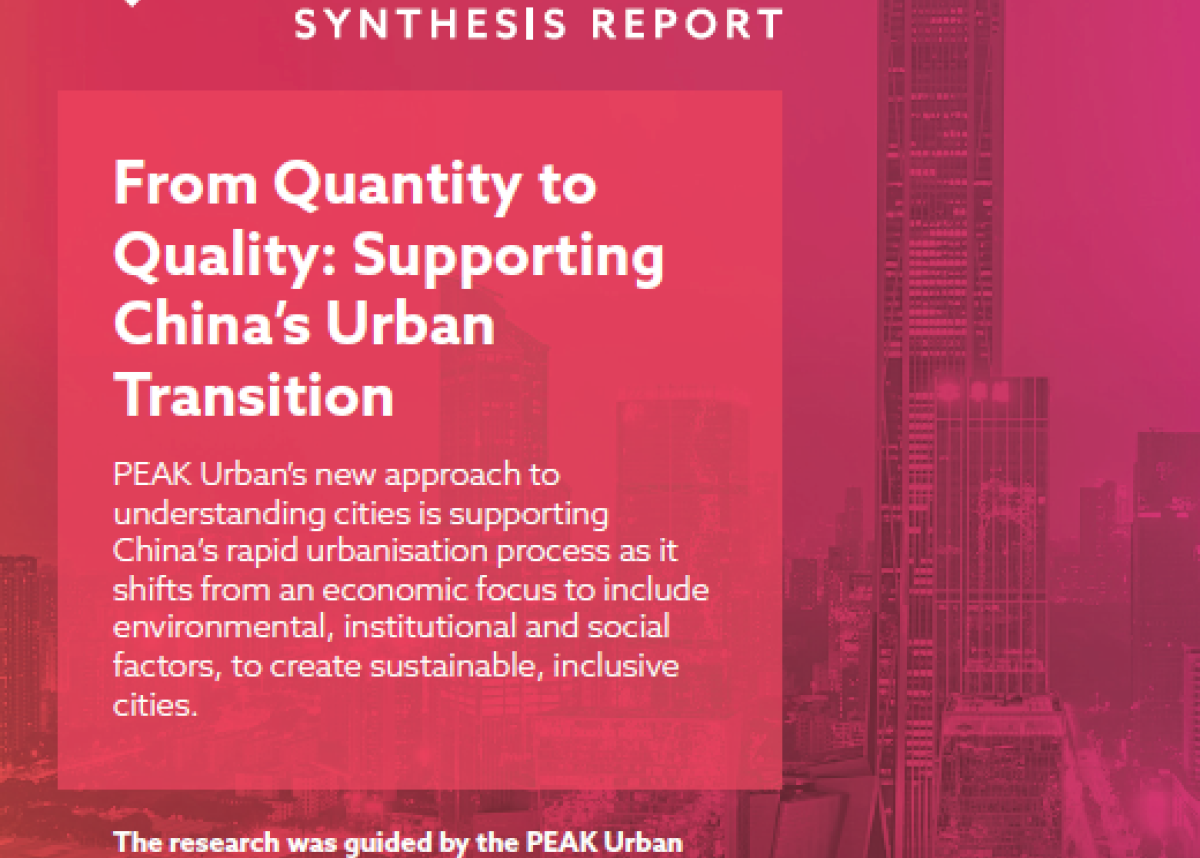
Identifying Metro Trip Purpose using Multi-source Geographic Big Data and Machine Learning Approach
Identifying the purpose of a metro trip using Smart Card Data (SCD) expands the application of SCD in transport research and transport planning.
This paper integrates different types of big data and combines the theories on the interaction between transport and land use.
By taking Beijing as a case, we firstly analyze the metro trip purposes of individual passengers using travel survey data from 5565 respondents. Secondly, we investigate the land use features of trip origin and destination using Point of Interest(POI) data . Thirdly, a metro trip dataset is developed which includes the information of trip purpose, trip duration, and spatial distribution of trip origin and destination. Fourthly, a Random Forest (RF) algorithm is used to establish a RF classifier using the metro trip dataset as training data. Finally, this trained classifier is used to classify each metro trip recorded by the SCD to identify the metro trip purpose and the spatial distribution of metro trips for different purposes.
The results of analysis show that the random forest classifier trained in this study can effectively identify metro trip purposes from SCD. For trips with "go to work" and "go home" purposes, the accuracy of identification can reach over 90%. One reason for the high identification accuracy is that land use information is included in the RF classifier.
Our results confirm the theory of spatial-temporal interactions between transport and land use. There is an increasing availability of multi-source geographic big data and traffic survey data of residents in large cities, which means that the method developed in this study would have a high value in metro trip predicting and monitoring, transport planning, and land use policy-making around the metro stations. Also, our results enhance our knowledge of metro travel behavior in megacities.